Convolutional neural network diagnostic performance appears to be comparable to that of radiologists for intracranial haemorrhage (ICH)
The use of convolutional neural networks (CNN) for the diagnosis of patients with an intracranial haemorrhage (ICH) appears to be comparable to that of radiologists according to a study by a team from the Faculty of Health and Medical Sciences, Copenhagen University, Denmark.
An ICH usually occurs due to a rupture of small penetrating arteries secondary to hypertensive changes or other vascular abnormalities and accounts for 10 – 20% of all strokes. However, this proportion varies across the world so that in Asian countries, an ICH is responsible for between 18 and 24% of strokes but only 8 – 15% in Westernised countries. An acute presentation of ICH can be difficult to distinguish from ischaemic stroke and non-contrast computerised tomography (CT) is the most rapid and readily available tool for the diagnosis of ICH.
The growth of artificial intelligence in medicine has advanced significantly in recent years and one such development is a Convolutional Neural Network (CNN), which is a Deep Learning algorithm that can take an input image, assign importance to various aspects or objects within in the image and to differentiate one from the other. In fact, a 2019 systematic review of Deep Learning systems concluded that the ‘diagnostic performance of deep learning models to be equivalent to that of health-care professionals.’ Nevertheless, the authors added the caveat that ‘few studies presented externally validated results or compared the performance of deep learning models and health-care professionals using the same sample.’
In the present study, the Danish researchers undertook a systematic review and meta-analysis to appraise the evidence of CNN in per-patient diagnosis of ICH. They performed a literature review and studies deemed suitable for inclusion were those where: patients had undergone non-contrast computed tomography of the cerebrum for the detection of an ICH; radiologists or a clinical report was used as the reference standard and finally where a CNN algorithm was deployed for the detection of ICH. For the purposes of their analysis, the minimum acceptable reference standard was defined as either manual, semi-automated or automated image labelling taken from radiology reports or electronic health records. For their analysis, the researchers calculated the pooled sensitivity, specificity and the receiver operating characteristics curves (SROC).
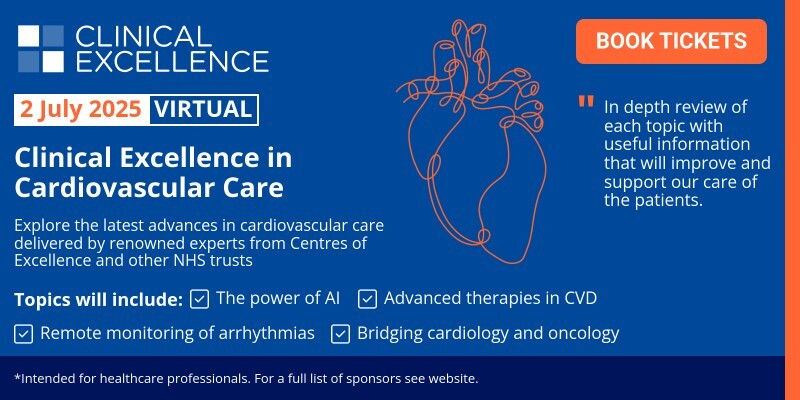
Findings
A total of six studies with 380,382 scans were included in the final analysis. When comparing the CNN performance to the reference standard, the pooled sensitivity was 96% (95% CI 93 – 97%), pooled specificity 97% (95% CI 90 – 99%) and an SROC of 98% (95% CI 97 – 99%). When combining both retrospective and external validation studies, for CNN, the performance was slightly worse with a pooled sensitivity of 95%, specificity 96% and pooled SROC 98%.
They concluded that CNN-algorithms can accurately detect ICHs based on an analysis of both retrospective and external validation studies and that this approach seemed promising but highlighted the need for more studies using external validation test sets with uniform methods to define a more robust reference standard.
Citation
Jorgensen MD et al. Convolutional neural network performance compared to radiologists in detecting intracranial hemorrhage from brain computed tomography: A systematic review and meta-analysis. Eur J Radiol 2021